Towards Zero Waste and Failures: AdditiveLab’s Customizable Simulation Enables Increase in Metal Additive Manufacturing Efficiency
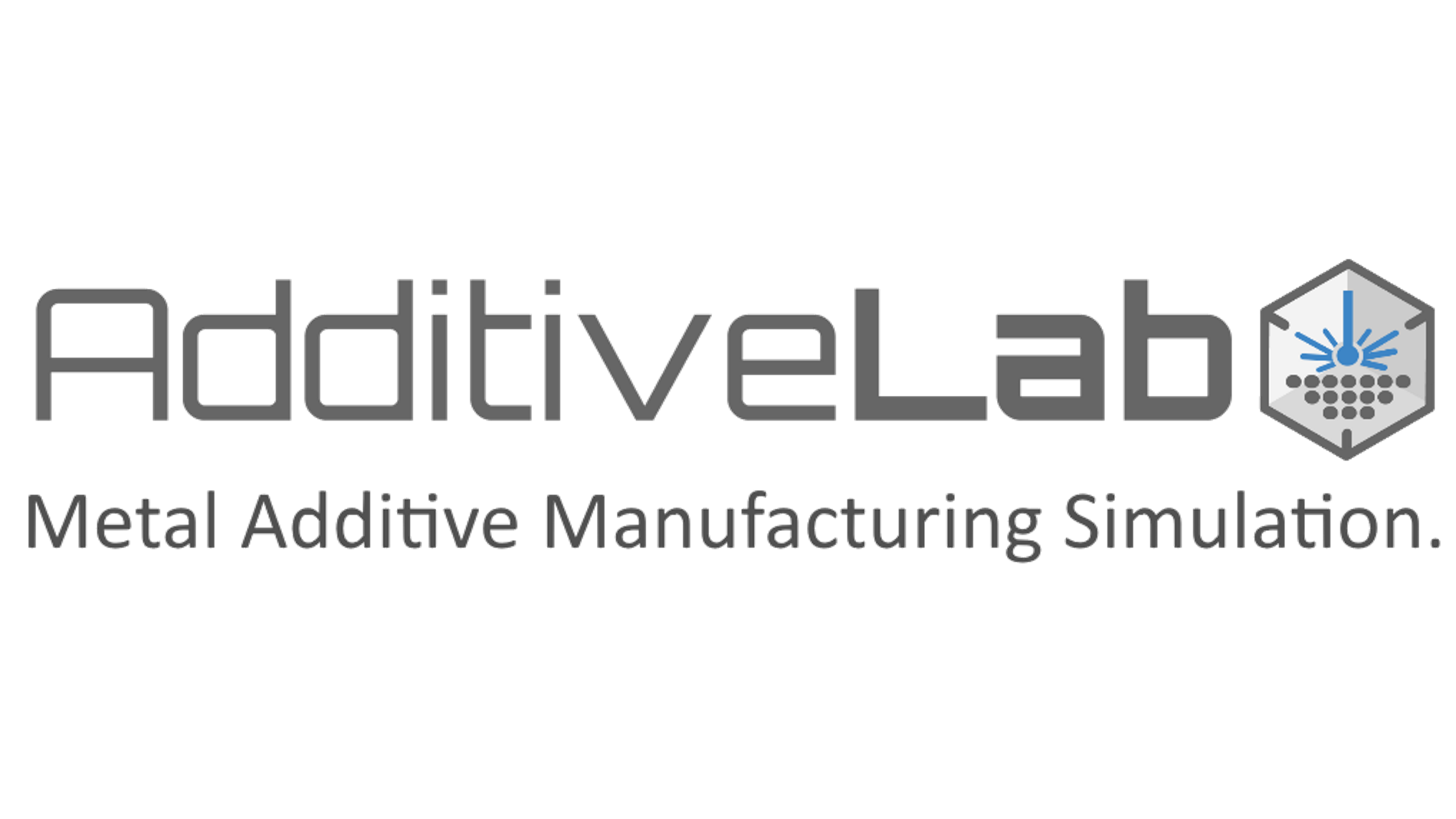
AdditiveLab’s Mariam Mir will be speaking at 3DPrint.com’s upcoming AMS online industry summit (Feb 9-10, 2021). Register here.
Metal additive manufacturing (AM) process simulation predicts the potential production outcome and gives vital information on regions of manufactured designs that are prone to deformations, high stresses and heat accumulation. With more insight into potential manufacturing outcomes entire build configurations including part and support structures can be optimized to increase the process efficiency and to minimize manufacturing risks.

The predicted model in AdditiveLab indicates the regions in red as the region prone to the most deformation.
Simulation for metal AM processes offers clear advantages to users, however, most simulation tools used in industry are designed to force the user to employ software specific simulation workflows. On the one hand, that makes it easy for users to quickly adapt the relatively complex simulation technology but on the other hand, due to the fixed workflow’s rigidity, advanced users are limited in creating customized simulation models which fit their exact needs. With such rigid simulation tools, advanced simulation users are not allowed detailed access to the simulation models and intermediate simulation results, and actual simulation parameters are often hidden from the user and are not manipulatable. This restricts users from adjusting the simulation models to better correlate with their production process. The opportunities for using intermediate data to extract conclusions, the possibility to create automated simulation workflows for various manufacturing scenarios and the possibility to create Intellectual Property (IP) within their enterprise are often not feasible due to these limitations.
The software of AdditiveLab addresses these AM simulation needs; their product AdditiveLabRESEARCH is specifically designed for advanced users allowing them to create simulation models with high level of simulation customization. This is achieved by giving the user access to in-depth AM simulation functionality via a powerful, integrated Python Application Programming Interface called the “AdditiveLab Python API”. The AdditiveLab Python API allows users access to all underlying software functions and enables them to create customization of simulation models, development of new simulation models, automation of customized simulation workflows and subsequently creation of their own simulation IP, which can then be easily shared within the user’s organization. Users are able to access all available simulation results at every existing simulation step and can export them in various formats to other software tools.
To elaborate the flexibility of creating optimizations using the AdditiveLab Python API, take the example of support optimization from one of AdditiveLab’s users from the dental industry. This customer case illustrates 40% support material reduction with optimization via the AdditiveLab Python API. The below illustrated part support configuration of the dental implant was generated with an established workflow at the user’s site that utilizes sturdy support structures in order to ensure failure-free manufacturing for a broad variety of dental implants on a daily basis. The supports are over-dimensioned to ensure daily production success, but subsequently, require more material usage, increased manufacturing times and longer manual post-processing. Even though the customer had their limitations in modifying the established workflow, they wanted to optimize existing support thickness to reduce material waste, machine runtimes and post-processing efforts to reduce costs.

The figure above shows the originally designed support for the dental implant with the over-dimensioned, cone-like support structures.
The customer employed the AdditiveLabRESEARCH software to determine which are the essential supports that are exposed to higher tension loads that could lead to failures during the production and compromisation of the manufacturing quality. During the AM process, residual stresses deform the part-support configuration which must be counteracted by the supports. Thus, it is vital to understand first which supports are exposed to higher loading and which ones are exposed to lesser loading and may have potential for volume reduction.
Process simulations immediately indicate that different cones were exposed to different loads (tensions) as illustrated in the images below. With further analysis, the reaction forces occurring in the cone supports due to the tension loads are used as a measure to optimize the cone structures.
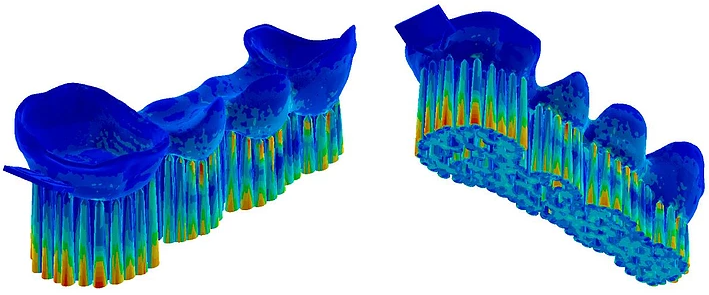
The figures above show the simulation results of the building process. The color-coding shows the tension loads at the bottom of the support structure with red indicating higher tension loads.
After understanding the effect of the loads occurring on the cones during the building process, the cone diameter was iteratively optimized based on the tension loads via simulation algorithms written in the AdditiveLab Python API. One example result of the optimization with this algorithm is illustrated in the figures below:
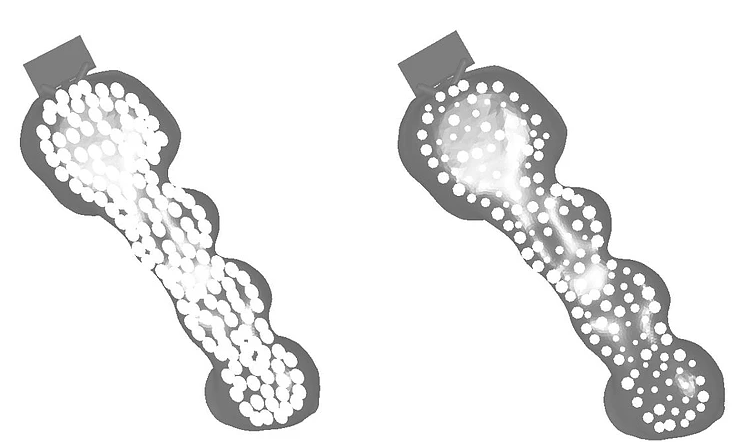
The figures above show the bottom view of the dental design with the originally designed support structures (left) and the optimized structures (right). The difference between the cone support diameters is illustrated with the optimized design needing 40% less material without compromising the structural integrity during the building process.
This project illustrates a real case example where the AdditiveLab Python API was used to enable the user with customizable, beyond standard simulation features. In this example the particular challenge was to utilize simulation around an established workflow allowing the customer to reduce material waste without increasing the build failure risk. The customized simulation aided greatly in better performance of the AM process for the dental implant manufacturer.
With using the AdditiveLab Python API, advanced simulation users, researchers and AM teams have the power at their disposal to create customized simulations, to optimize their processes and to create their own simulation IP.
Discover more about AdditiveLabRESEARCH and AdditiveLab automated and simplified AdditiveLabLITE solution on our website and join us at AMS conference in the panel session 2, Design Software for AM to learn more about additive manufacturing simulation and other software tools you need for additive manufacturing.
Subscribe to Our Email Newsletter
Stay up-to-date on all the latest news from the 3D printing industry and receive information and offers from third party vendors.
Print Services
Upload your 3D Models and get them printed quickly and efficiently.
You May Also Like
3D Printing News Briefs, July 2, 2025: Copper Alloys, Defense Manufacturing, & More
We’re starting off with metals in today’s 3D Printing News Briefs, as Farsoon has unveiled a large-scale AM solution for copper alloys, and Meltio used its wire-laser metal solution to...
Etsy Design Rule Change Reduces Selection of 3D Printed Goods
Online marketplace Etsy has implemented a rule change requiring all 3D printed goods on the site to be original designs. The update to the site’s Creativity Standards states, ¨Items produced using...
Siraya Tech Introduces New Elastomer 3D Printing Materials, Including Foaming TPU
California company Siraya Tech, founded in 2019 with a focus on material science, customer focus, and agility, develops high-quality 3D printing materials that meet the needs of creators, hobbyists, and...
3D Printing News Briefs, April 12, 2025: RAPID Roundup
The news from last week’s RAPID+TCT in Detroit just keeps on coming! That’s why today’s 3D Printing News Briefs is another RAPID Roundup of more exciting announcements from the trade...