Researchers at Rice University used artificial intelligence (AI) to speed up the development of 3D printed bioscaffolds that help injuries heal. A team led by computer scientist Lydia Kavraki of Rice University’s Brown School of Engineering used two machine learning approaches to predict the quality of scaffold materials, given the printing parameters. Results from the study published in the journal Tissue Engineering Part A found that controlling print speed is critical in making high-quality implants.
Thanks to the accurate data processing and prediction capabilities of AI, scientists in biotechnology are extensively relying on AI tools and techniques – like machine and deep learning – to solve actual problems and improve discoveries that will aid disease diagnosis and treatment, personalized medicine, and more.
According to a report by PricewaterhouseCoopers, AI could contribute up to $15.7 trillion to the global economy in 2030, while a recent survey of pharma and life sciences experts showed that 44% were already using AI in their research and development activities.

A “high quality” 3D-printed bioscaffold as designed with help from a machine learning algorithm developed at Rice University. Scale bar equals 1 millimeter. (Image courtesy of the Mikos Research Group)
Rice University bioengineer and study co-author Antonios Mikos has been developing bioscaffolds, largely with the Center for Engineering Complex Tissues, to improve techniques to heal craniofacial and musculoskeletal wounds. According to Rice University, the bioscaffolds are bonelike structures that serve as placeholders for injured tissue. They are porous to support the growth of cells and blood vessels that turn into new tissue and ultimately replace the implant.
Mikos’ work has progressed to include sophisticated 3D printing that can make a biocompatible implant custom-fit to the site of a wound. Now, with the help of machine learning techniques, designing materials, and developing processes to create implants can be faster and eliminate much trial and error.
The team explored two machine learning-based modeling approaches. One was a direct classification-based method that predicted whether a given set of parameters would produce a “low” or “high” quality scaffold print. The other was a regression-based approach that approximated the values of a printing-quality metric to come to a result. The researchers reported that both models are built upon a “classical supervised learning technique” called random forests that builds multiple “decision trees” and “merges” them together to get a more accurate and stable prediction.
The team trained and evaluated the models on a dataset generated in a previous study that investigated the fabrication of porous polymer scaffolds by means of extrusion-based 3D printing with a full-factorial design. Ultimately, this collaboration could lead to better ways to quickly print a customized jawbone, kneecap or bit of cartilage on demand.
“A hugely important aspect is the potential to discover new things. This line of research gives us not only the ability to optimize a system for which we have a number of variables – which is very important – but also the possibility to discover something totally new and unexpected. In my opinion, that’s the real beauty of this work,” said Mikos, the Louis Calder Professor of Bioengineering and Chemical and Biomolecular Engineering and a professor of chemistry and materials science and nanoengineering at Rice University. “It’s a great example of convergence. We have a lot to learn from advances in computer science and artificial intelligence, and this study is a perfect example of how they will help us become more efficient.”
Mikos and his students had already considered bringing machine learning into the mix, but the 2020 COVID-19 pandemic created a unique opportunity to pursue the project. From start to finish, the COVID-19 window allowed them to assemble data, develop models, and get the results published within seven months, which is considered record time for a process that can often take years, explained Rice University. Although the students had to figure out how to communicate with each other remotely, once they did, they advanced really quickly. For Mikos, this was a way to make great progress at a time when many students and faculty were unable to go to the lab.
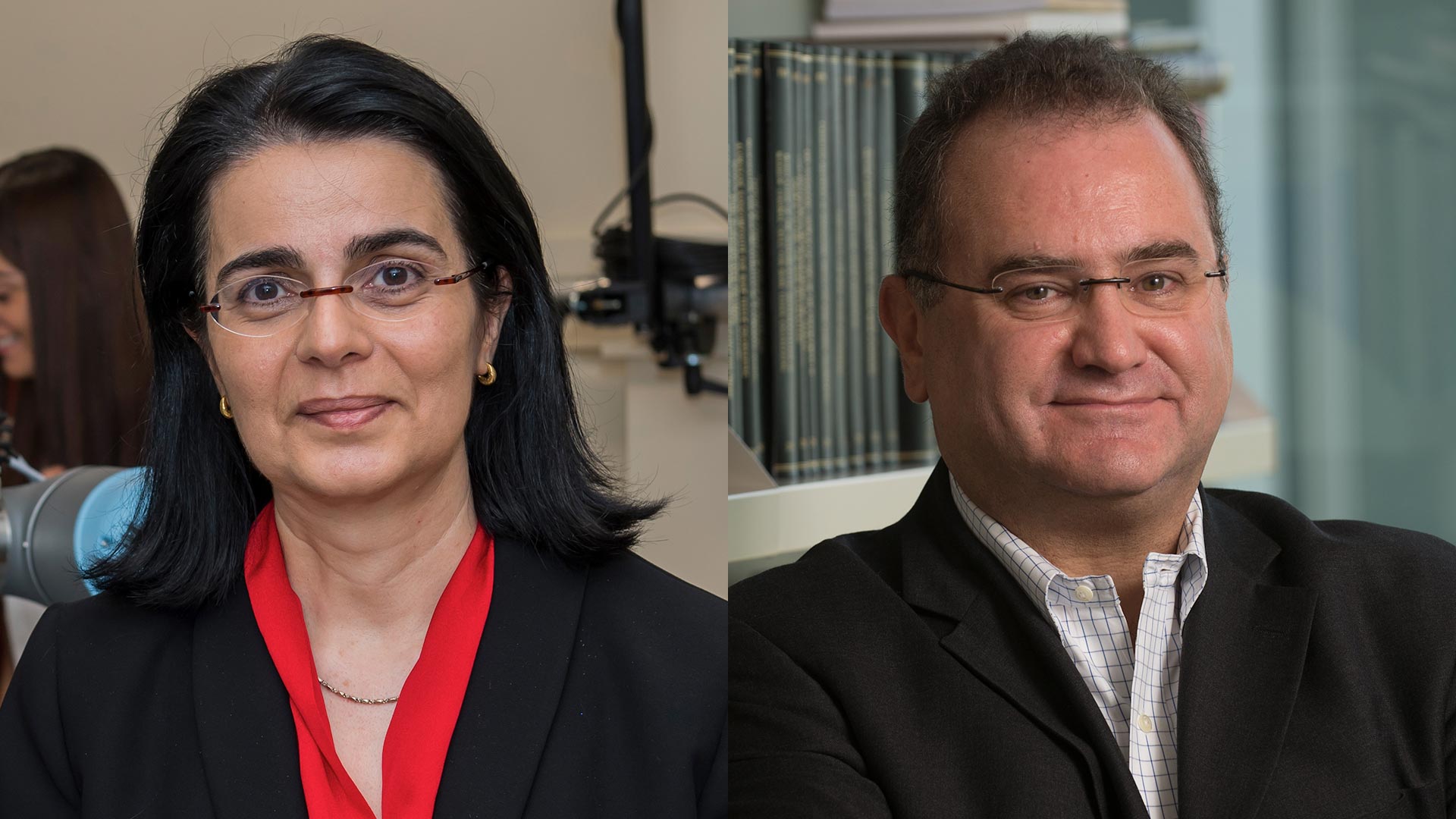
Rice computer scientist Lydia Kavraki and Rice bioengineer Antonios Mikos. (Image courtesy of Rice University’s Brown School of Engineering)
Kavraki said the researchers and co-authors of the paper—graduate students Anja Conev and Eleni Litsa in her lab and graduate student Marissa Perez and postdoctoral fellow Mani Diba in the Mikos lab—took time at the start to establish an approach to a mass of data from a 2016 study on printing scaffolds with biodegradable poly(propylene fumarate) (PPF), and then to figure out what more was needed to train the computer models. The study identified print speed as the most important of five metrics the team measured, the others in descending order of importance were material composition, pressure, layering, and spacing.
“We were able to give feedback on which parameters are most likely to affect the quality of printing, so when they continue their experimentation, they can focus on some parameters and ignore the others,” said Kavraki, a renowned authority on robotics, artificial intelligence and biomedicine and director of Rice’s Ken Kennedy Institute. “In the long run, labs should be able to understand which of their materials can give them different kinds of printed scaffolds, and in the very long run, even predict results for materials they have not tried. We don’t have enough data to do that right now, but at some point, we think we should be able to generate such models.”
Kavraki also noted that AI has a role to play in new materials, especially as there are so many problems at the intersection of materials science and computing, and the more people can get to work on them, the better. She also suggested that the recently established Welch Institute, at Rice University to enhance the institution’s “already stellar reputation for advanced materials science,” has great potential to expand collaborations that incorporate AI technology into bioengineering research.
Subscribe to Our Email Newsletter
Stay up-to-date on all the latest news from the 3D printing industry and receive information and offers from third party vendors.
You May Also Like
Further Understanding of 3D Printing Design at ADDITIV Design World
ADDITIV is back once again! This time, the virtual platform for additive manufacturing will be holding the first-ever edition of ADDITIV Design World on May 23rd from 9:00 AM –...
3D Printer Maker EVO-tech Reborn as NEVO3D — Once More With Feeling
EVO-tech was a 3D printing service and original equipment manufacturer established in 2013 and based in Schörfling am Attersee, Austria. The company produced high-quality material extrusion systems featuring linear bearings,...
3D Systems Brings 3D Printed PEEK Cranial Implant to the U.S. with FDA Clearance
For more than 10 years, 3D Systems (NYSE:DDD) has worked hand-in-hand with surgeons to plan over 150,000 patient-specific cases, and develop more than two million instruments and implants from its...
CDFAM Returns to Berlin for Second Annual Symposium
The second CDFAM Computational Design Symposium is scheduled for May 7-8, 2024, in Berlin, and will convene leading experts in computational design across all scales. Building upon the first event...