In ‘Applying Neural-Network-Based Machine Learning to Additive Manufacturing: Current Applications, Challenges, and Future Perspectives,’ authors Xinbo Qi, Guofeng Chen, Yong Li, Xuan Cheng, and Changpeng Li investigate how machine learning (ML) and neural network algorithms (NN) can be applied to additive manufacturing.
While the many benefits of AM processes continue to be uncovered, availing themselves to countless industries today, there are still numerous drawbacks and scenarios for defects which continue to challenge users around the world—from porosity to anisotropic microstructures, to distortion, and more.
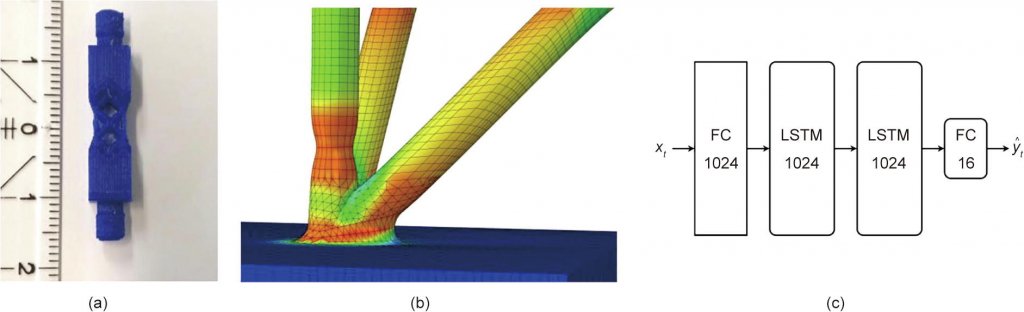
Application of an NN model to predict the deformation of an AM structure. (a) Specimens, which are manufactured and tested under controlled loading conditions; (b) the FEM, whose simulation results are validated by specimens; (c) the NN, which is trained by the data generated by the FEM, and then used to predict the deformation history in a faster way than the FEM. FC: Fully-connected layers. Reproduced from Ref. [31] with permission of Elsevier, © 2018.
- Powder’s metallurgical parameters
- 3D printing process
- Microstructure
- Mechanical properties of AM parts
In machine learning, the NN algorithm is only increasing in popularity for use and is currently under ‘rapid development,’ most often employed in computer vision, voice recognition, language processing, and self-driving vehicles. It is a supervised type of ML, operating with labeled data, and within additive manufacturing is showing good suitability for ‘agile manufacturing’ in industry.
“The NN has exerted a deep and wide impact on all value chain innovation in industry—from product design, manufacturing, and qualification to delivery—and it is believed that the impact of NN will be increasingly intensive,” state the researchers.
The most common types of NNs are:
- Multilayer perceptron (MLP)
- Convolutional neural network (CNN)
- Recurrent neural network (RNN)
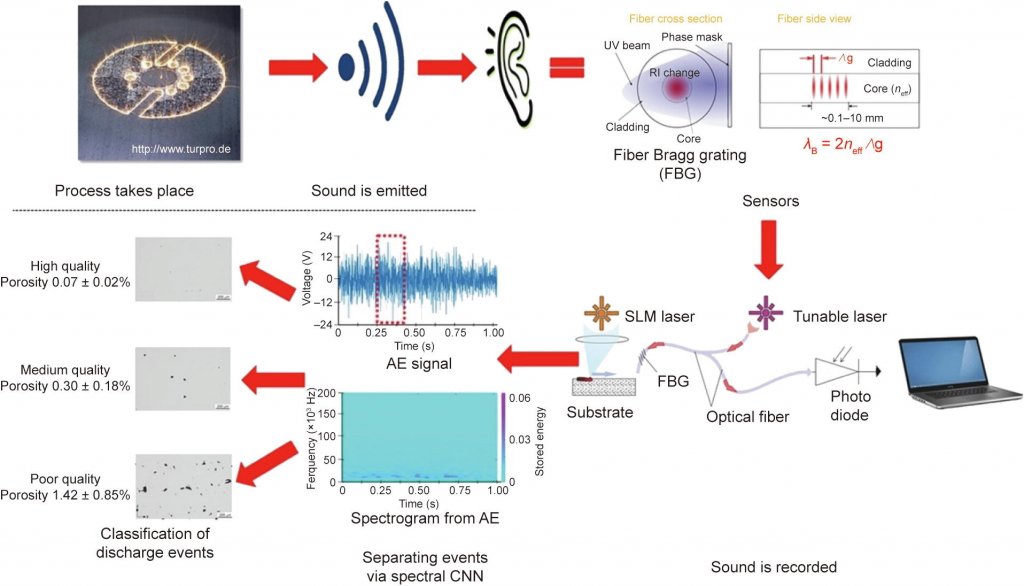
Scheme of the AM quality monitoring and analyzing system. The workflow is as follows: An acoustic signal is emitted during the AM process, and then captured by sensors; an SCNN model is finally applied to the recorded data in order to distinguish whether the quality of the printed layer is adequate or not. Reproduced from Ref. [35] with permission of Elsevier, © 2018.
Sensors have been created for the hardware and software, and a variety of different sensors can be used for in situ measurements too.
“The scope of this work covers many variants of NNs in various application scenarios, including: a traditional MLP for linking the AM process, properties, and performance; a convolutional NN for AM melt pool recognition; LSTM for reproducing finite-element simulation results; and the variational autoencoder for data augmentation. However, as they say, ‘every coin has two sides.’
“It is difficult to control the quality of AM parts, while NNs rely strongly on data collection. Thus, some challenges remain in this interdisciplinary area. We have proposed potential corresponding solutions to these challenges and outlined our thoughts on future trends in this field,” concluded the researchers.
Machine Learning is often connected with 3D printing, from varying monitoring methods and smarter metal additive manufacturing, to construction. What do you think of this news? Let us know your thoughts! Join the discussion of this and other 3D printing topics at 3DPrintBoard.com.
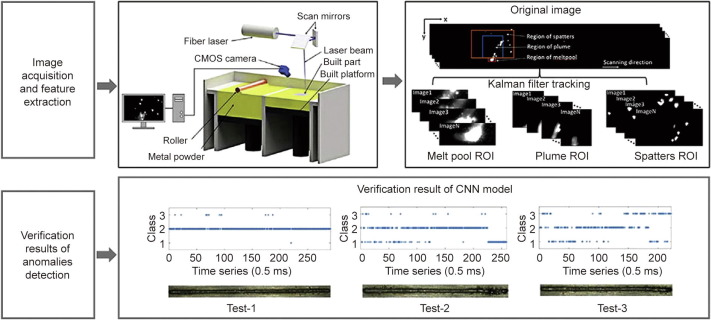
Scheme of the SLM process monitoring configuration. A high-speed camera is used to capture sequential images of the built process; a CNN model is applied to identify quality anomalies. ROI: region of interest. Reproduced from Ref. [37] with permission of Elsevier, © 2018.
Subscribe to Our Email Newsletter
Stay up-to-date on all the latest news from the 3D printing industry and receive information and offers from third party vendors.
You May Also Like
3D Printing Unpeeled: Biofuel Waste to Filament & Sustainable Photopolymers
I can’t ever remember a day with so many potentially high impact news stories have come out. In one story, we all know that there are problems with the safety...
Finnair Hires AM Craft to 3D Print Plastic Parts for Aircraft Interiors
Riga-based AM Craft, a supplier specialized in 3D printing aviation components and certified under EASA Part 21G, announced a significant achievement today. The company will assist in upgrading Finnair’s A320...
3DPOD Episode 198: High Speed Sintering with Neil Hopkinson, VP of AM at Stratasys
Neil Hopkinson, a pioneering 3D printing researcher, played a pivotal role in developing a body of research that is widely utilized today. He also invented High Speed Sintering (HSS), also...
3D Printing Webinar and Event Roundup: May 12, 2024
Webinars and events are picking up in the AM industry this week! ASTM International continues its Professional Certificate Course and Stratasys continues its advanced in-person trainings, while 3D Systems is...