Additive manufacturing (AM) continues to evolve in the dynamic manufacturing landscape, and integrating Artificial Intelligence (AI) has proven to be transformative. Both have independently made waves, redefining what’s possible in their respective domains. But it’s their convergence that promises a manufacturing revolution.
This article will explore the intersection of AI and 3D printing. We’ll particularly shed light on how generative AI enhances the capabilities of AM, from optimizing designs to quality control needs. We will see an example that showcases the synergy between generative AI and 3D printing. Lastly, we will discuss innovators’ challenges in this field while moving forward.
How AI Enhances AM
Artificial Intelligence (AI) is transforming AM with enhancements and tailored methods:
- Generative Design: AI’s capability in design optimization is remarkable. Given initial constraints, AI explores myriad design variations, yielding lighter, more robust, and more efficient parts than those humanly conceptualized.
- Process Optimization: AI fine-tunes the manufacturing process to guarantee consistency in print quality. AI ensures optimal printing conditions by adjusting parameters like print speed, layer height, or temperature based on sensor feedback.
- Machine Learning for Quality Control: AM’s quality assurance benefits from AI’s analytical eye. Advanced machine learning models, such as Convolutional Neural Networks (CNNs), assess images of printed components to identify defects or design deviations.
- Predictive Maintenance: Leveraging AI for analyzing operational data allows for preemptive equipment maintenance. This reduces unplanned downtime, ensuring the manufacturing process is smooth and efficient.
- Material Innovations: AI’s prowess extends to material selection and innovation. It assists in picking suitable materials and suggests new combinations tailored for desired properties. Neural network models predict the characteristics of novel materials, providing insights even before their production.
- Reinforcement Learning for Process Refinement: Continuous improvement is vital in AM, and reinforcement learning aids this by taking actions that maximize outcomes like achieving unparalleled material properties or surface finishes.
- Documentation through NLP: As designs evolve, so should the documentation. AI employs Natural Language Processing (NLP) techniques to auto-update or create documentation in alignment with design changes.
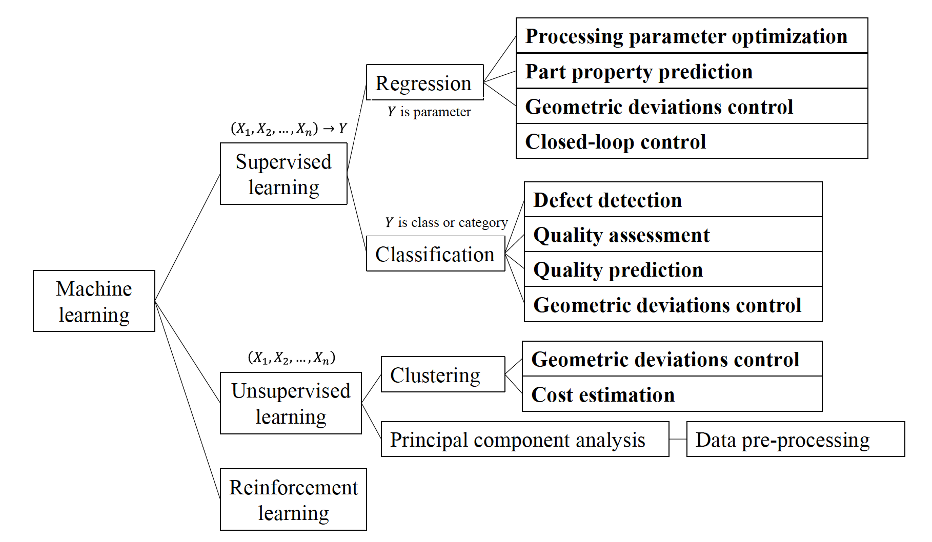
ML categories with possible applications in the AM field,
Meng, L., McWilliams, B., Jarosinski, W. et al. Machine Learning in Additive Manufacturing: A Review. JOM 72, 2363–2377 (2020). https://doi.org/10.1007/s11837-020-04155-y
The Rise of Generative AI and Its Implications
Generative AI is a type of AI that has the ability to generate new content or designs based on learned data patterns. This technology has brought manifold implications in the world of AM. Recently, ChatGPT demonstrated its impact in various fields, highlighting the transformative potential of generative AI in the manufacturing industry.
One of generative AI’s most notable advantages is its swift pace at which it facilitates design evolution. Rather than prolonged development cycles, this technology can rapidly produce multiple design options tailored to specific constraints, streamlining the journey from initial concept to final design and reducing the need for multiple prototyping iterations. An adventurous spirit of innovation accompanies this acceleration in design processing. Generative AI isn’t constrained by human biases or traditional design paradigms, allowing it to explore unique design avenues that may, at first glance, seem counterintuitive but have the potential to lead to innovative solutions.
Beyond mere design, generative AI’s prowess extends to customization on an unprecedented scale. Whether it’s fashioning prosthetics meticulously tailored to an individual’s unique physiology or engineering car components designed to perform optimally in specific environmental conditions, generative AI places personalization at the epicenter of manufacturing. This commitment to precision doesn’t come at the expense of sustainability or efficiency. By optimizing designs, generative AI ensures products are crafted using the least possible material, marrying strength and functionality without wastage. Such resource efficiency not only champions sustainable manufacturing practices but also proves to be cost-effective.
Seamless integration with 3D printing processes is another feather in generative AI’s cap. Its inherently digital-first designs transition smoothly into the AM world, guaranteeing that the physical manifestation of products aligns perfectly with their digital blueprints. Even more intriguing is that generative AI isn’t static; it’s perpetually evolving. With every feedback loop, from user responses to performance metrics and newly discovered material properties, it hones its design suggestions, making them more sophisticated and closely aligned with real-world requirements.
As we stand at this juncture, the marriage of generative AI and AM not only promises but also delivers a future where designs are efficient, customizable, and continuously evolving, reshaping the very fabric of the manufacturing industry.
PIX Moving, using Generative AI for Automotive Design
PIX Moving, a Chinese startup, uses generative AI uniquely to reimagine the automotive industry. They have developed a decentralized method of automotive manufacturing that leverages generative design, robotics, and 3D printing.
In their Generative Design Hackathon, PIX Moving aimed to explore new automobile manufacturing paradigms with creative digital design approaches and new digital manufacturing processes. The ultimate goal of the hackathon was to design self-driving cars that can meet 3D printing capacities using a generative design approach.
In another initiative, the Moving Space Hackathon, PIX Moving launched a global design competition to reimagine autonomous rideshare vehicles as dual-purpose spaces. For this competition, participants were granted exclusive access to PIX Moving’s patented generative design algorithm PAM, which they describe as the “ChatGPT of car design and manufacturing – interior and exterior.” This shows how PIX Moving pushes the boundaries of generative AI in automotive design and manufacturing.
PIX Moving is envisioning a future where innovative designers create car designs in a way that hasn’t been considered before. They are reducing the structural parts of a traditionally manufactured electric car to 10 parts and manufacturing the car with 3D printing technology.
Navigating Challenges
While the convergence of generative AI and AM holds promise for revolutionary designs and production methods, it’s not without challenges. Recognizing these challenges and developing strategies to address them will be key to harnessing the full potential of this integration.
- Computational Demands: Generative AI models can be computationally intensive, especially when dealing with complex geometries and constraints. Organizations might need to invest in high-performance computing clusters or leverage cloud platforms.
- Data Quality and Availability: The effectiveness of generative AI is often contingent on the quality and quantity of data it’s trained on. Insufficient or biased data can lead to suboptimal or even flawed output.
- Interpreting AI Outputs: Sometimes, the designs generated by AI might be counterintuitive or unconventional. Engineers and designers must critically assess these outputs, ensuring they align with safety standards and practical considerations.
- Integration with Existing Systems: Introducing AI-driven design processes might require significant changes in existing design and manufacturing workflows. Seamless integration will be crucial to avoid disruptions.
- Material Limitations: While AI might suggest intricate and novel designs, the current range of materials suitable for 3D printing could limit their real-world applicability.
- Intellectual Property Concerns: Determining ownership and patentability of AI-generated designs can be a legal gray area. Companies need to navigate these challenges to protect their innovations.
- Reliability and Testing: AI-designed products need rigorous testing, especially in critical applications like aerospace or medical devices. Traditional testing protocols might need to be adapted or expanded.
- Cost Implications: While generative AI can lead to cost savings in material and design optimization, the initial investment in AI infrastructure, training, and integration could be substantial.
- Human-AI Collaboration: The optimal use of generative AI isn’t to replace human designers but to augment their capabilities. Establishing a collaborative framework where humans and AI work together can yield the best results.
- Regulatory and Certification Challenges: Products, especially in regulated industries, must meet specific standards. Regulators might need to adapt their frameworks to accommodate and validate AI-generated designs.
Conclusion
As we look ahead, the coalition of generative AI and 3D printing signals towards a future where the boundaries of design and production are constantly expanded and redefined. The synergistic relationship between AI and AM is transformative and symbolic of a future where technology is intricately intertwined with inventive manufacturing. However, it is imperative that as we navigate through this innovative journey, we approach the challenges with strategic forethought, ensuring that this technological symbiosis does not just innovate but also sustains and evolves, ever propelling towards a boundlessly innovative future.
Subscribe to Our Email Newsletter
Stay up-to-date on all the latest news from the 3D printing industry and receive information and offers from third party vendors.
You May Also Like
Further Understanding of 3D Printing Design at ADDITIV Design World
ADDITIV is back once again! This time, the virtual platform for additive manufacturing will be holding the first-ever edition of ADDITIV Design World on May 23rd from 9:00 AM –...
3D Printer Maker EVO-tech Reborn as NEVO3D — Once More With Feeling
EVO-tech was a 3D printing service and original equipment manufacturer established in 2013 and based in Schörfling am Attersee, Austria. The company produced high-quality material extrusion systems featuring linear bearings,...
3D Systems Brings 3D Printed PEEK Cranial Implant to the U.S. with FDA Clearance
For more than 10 years, 3D Systems (NYSE:DDD) has worked hand-in-hand with surgeons to plan over 150,000 patient-specific cases, and develop more than two million instruments and implants from its...
CDFAM Returns to Berlin for Second Annual Symposium
The second CDFAM Computational Design Symposium is scheduled for May 7-8, 2024, in Berlin, and will convene leading experts in computational design across all scales. Building upon the first event...