Researchers from the Daniel J. Epstein Department of Industrial and Systems Engineering, led by Qiang Huang, Ph.D., have recently been granted $1.4M funding support, including a recent $350,000 NSF grant. Assisted by Ph.D. students Yuanxiang Wang, Nathan Decker, Mingdong Lyu, Weizhi Lin and Christopher Henson, as the team continues to research better ways to streamline 3D printing—making the technology ‘smarter’ with machine learning—they have released numerous articles on the subject.
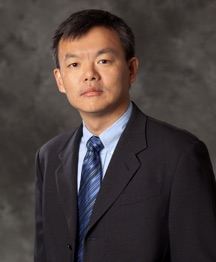
Qiang Huang, Ph.D., Associate Professor of Industrial and Systems Engineering and Chemical Engineering and Materials Science
While the benefits of 3D printing are being made obvious on a global level—and from users who range from beginners to serious industrialists—challenges are still so numerous that researchers stay busy working out the kinks, as well as taking the technology to new levels due to requirements that arise in countless innovative projects.
In the research team’s latest published work on the subject, ‘Shape Deviation Generator—A Convolution Framework for Learning and Predicting 3D Printing Shape Accuracy,’ the authors explain how machine learning for AM (ML4AM) has become realistic for use in improving 3D printing processes:
“The physics-based modeling and simulation approaches present voxel-level description of an object formation from points to lines, lines to surfaces, and surfaces to 3D shapes. However, this computationally intensive modeling framework does not provide a clear structure for machine learning of AM data,” state the researchers in their abstract. “Significant progress has been made to model and predict the shape accuracy of planar objects under data analytical frameworks.”
The goal is to lessen errors; for example, shape distortion causes many prints to fail, thus resulting in discards—and depending on the material, it could mean a lot of waste. With PrintFixer, a new software tool created by the researchers, machine-learning algorithms can improve digital fabrication accuracy by up to 50 percent or more.
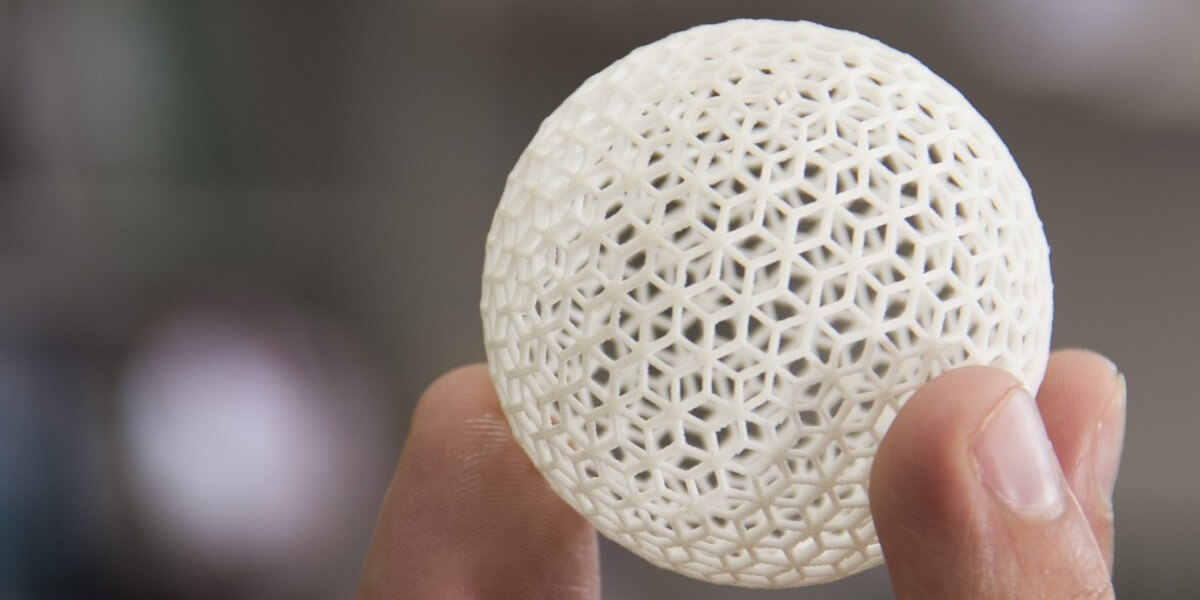
PrintFixer is a new machine learning driven software package that will make 3D printing 50 percent more accurate (Image: PXHERE)
“What we have demonstrated so far is that in printed examples the accuracy can improve around 50 percent or more,” Huang said. “In cases where we are producing a 3D object similar to the training cases, overall accuracy improvement can be as high as 90 percent.
“It can actually take industry eight iterative builds to get one part correct, for various reasons,” Huang said, “and this is for metal, so it’s very expensive.”
The key is to deviate as little as possible from the original design, meaning that better control must be maintained over hardware and materials. With machine learning processes, software like PrintFixer uses data from previous jobs to ‘understand’ and prevent future errors before they happen.
“From just five to eight selected objects, we can learn a lot of useful information,” Huang said. “We can leverage small amounts of data to make predictions for a wide range of objects.”
The researchers have tested their software successfully and have used it in a range of applications, with varied materials—to include commercial thermoplastics and metals in aerospace projects.

A screenshot from PrintFixer shows the predicted variations in a printed shape, with expanded areas highlighted in red and smaller areas marked in blue.
“So just like a when a human learns to play baseball, you’ll learn softball or some other related sport much quicker,” said Nathan Decker, acting as software development lead. “In that same way, our AI can learn much faster when it has seen it a few times.”
Users can also use the software to predict whether another 3D printer of their choice—and of better quality—would give them improved results.
“But if you don’t want to change the printer, we also have incorporated functionality into the software package allowing the user to compensate for the errors and change the object’s shape – to take the parts that are too small and increase their size, while decreasing the parts that are too big,” said Decker. “And then, when they print, they should print with the correct size the first time.”
Now, the group is working with a dental clinic in Australia, expanding their technology for 3D printing dental models. Their continued goal, however, is to make the software accessible to everyone—on all levels.
“Say I’m working with a MakerBot 3D printer using PLA (a bioplastic used in 3-D Printing), I can put that in the database, and somebody using the same model and material could take my data and learn from it,” Decker said.
“Once we get a lot of people around the world using this, all of a sudden, you have a really incredible opportunity to leverage a lot of data, and that could be a really powerful thing,” he said.
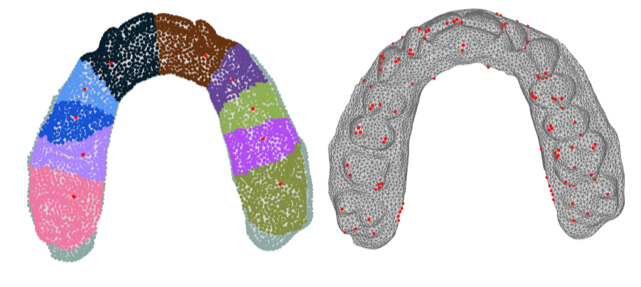
Ph.D. student Weizhi Lin’s work is ensuring 3D printed models of dental patients’ teeth are accurately matched to their designs. The red points on the model are automatically-selected landmarks to ensure the complex shape can be printed accurately.
Machine learning continues to be integrated into 3D printing practices, from the use of neural networks to experimenting with soft materials, bioprinting, and more. What do you think of this news? Let us know your thoughts! Join the discussion of this and other 3D printing topics at 3DPrintBoard.com.
[Source / Images: USC Viterbi School of Engineering; ‘Shape Deviation Generator—A Convolution Framework for Learning and Predicting 3D Printing Shape Accuracy’]Subscribe to Our Email Newsletter
Stay up-to-date on all the latest news from the 3D printing industry and receive information and offers from third party vendors.
You May Also Like
Further Understanding of 3D Printing Design at ADDITIV Design World
ADDITIV is back once again! This time, the virtual platform for additive manufacturing will be holding the first-ever edition of ADDITIV Design World on May 23rd from 9:00 AM –...
3D Printer Maker EVO-tech Reborn as NEVO3D — Once More With Feeling
EVO-tech was a 3D printing service and original equipment manufacturer established in 2013 and based in Schörfling am Attersee, Austria. The company produced high-quality material extrusion systems featuring linear bearings,...
3D Systems Brings 3D Printed PEEK Cranial Implant to the U.S. with FDA Clearance
For more than 10 years, 3D Systems (NYSE:DDD) has worked hand-in-hand with surgeons to plan over 150,000 patient-specific cases, and develop more than two million instruments and implants from its...
CDFAM Returns to Berlin for Second Annual Symposium
The second CDFAM Computational Design Symposium is scheduled for May 7-8, 2024, in Berlin, and will convene leading experts in computational design across all scales. Building upon the first event...