Machine learning is a very hot topic right now, with ChatGPT causing quite a stir. Commercial company OpenAI released the chatbot on top of its language models. Repurposed for fun pictures and to write homework, ChatGPT is allowing the world to come to grips with what machine learning, often called artificial intelligence (AI) can do.
AI developers share the goal of making machines that “think” and, in so doing, pass as or equal the thought processes of human or other beings. The thoughts of such entities themselves could be very different from our own, but could be equal in speed or ability. In machine learning, a computer program uses rules, perhaps user inputs and data, to sort, sift, or make predictions about future data or outcomes.
Hundreds of thousands of pictures of dogs, labeled with the name of the animal and its breed, might be perused by the AI, eventually allowing the program to divine that German Shepherds are more likely to be called “Rex.” It might determine that Malamutes and Beagles are more likely to have heterochromia and do so without having access to specific dog Information, or even without knowing what eyes, heterochromia, or dogs even are. When these programs are married to artificial neural networks (a simulation of an animal brain), patterns can be found that we may not have foreseen or that we don’t have the time to figure out.
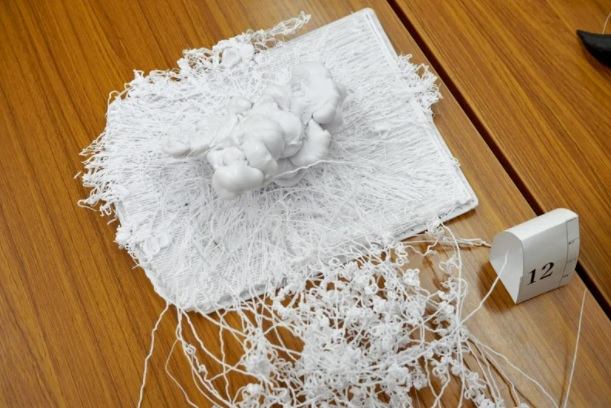
Undoubtedly, AI will be a powerful tool. What kind of impact will it have on the world? Of that, we cannot be sure. Nor can we precisely know in what time frame AI will percolate through to our jobs, daily lives, and economy. With the adoption of ChatGPT by AIBuild, we can see that 3D printing could really harness these tools today. However, what can AI do to the additive manufacturing industry and what is it likely to do in the near term? With regards to what we know now, we can make some inferences.
Settings Divination
On desktop material extrusion systems, finding the optimal settings for a filament is driven in part by experience, part by skill, and part by guess work. When machine manufacturers optimize parameters, they often do so through repeated test printing and adjustment based on their own initial settings. Filament manufactures dial in their feedstocks on a few printers sold by major brands, as well. Often users optically determine what is the best looking result, but more rigorous testing is on the rise.
Based on enough information, this process could be automated with AI. Given enough initial testing and results, a materials firm could input its changed formula and obtain an idea for the optimal settings of the new filament on many machines. By extending its testing to new systems, the company could have much better settings and print results than other filament vendors.
Another example could see an original equipment manufacturer look through all of its testing outcomes on the machine, materials, mass specifications, and more. It could then plot the settings and perceived results to come up with much better parameters per filament. It could then also optimize settings for higher strength for example with further testing.
Part Improvement
Strength testing different infill percentages and patterns should help an AI determine the optimal infill pattern, with different densities and settings further explored to provide the optimal configurations for different parts. The same can be done for layer adhesion settings, track thickness, and layer height to improve Z strength with better settings.
Fault Mitigation
A paper already published discusses how to detect surreptitious infill tampering using machine learning. Automated fault detection is something that has been discussed in a few papers. In this article, shapes are analyzed to see if they are correct. This journal paper discusses a system that has been designed to pass or fail good or bad 3D prints.
If the Spaghetti Detective or AstroPrint analyzed all of their video they could perhaps find common fault modes or occurrences, including particular heights, shapes, movements, and models that cause errors. Further access to user settings (or correlation with Cura) could generate an extremely large dataset of failed prints. This could then be used to avoid common errors or figure out the correct settings.
Process Monitoring
In a similar vein, process monitoring could detect errors, misprints, and other errors as early as possible, as discussed in numerous research articles and even pursued by a number of 3D printing companies. Over time indications, designs, support structures could be used to predict when and how prints will fail. Companies like Addiguru and Open Additive are already exploring these possibilities, as are Hexagon, Authentise, and many more.
A lot of the drudgery will be removed from quality assurance and in-process monitoring that can be used to make the entire system more predictive. This may lead to new understanding of feedback loops or the influence of certain variables on the print process.
Design Optimization
Designs themselves might be optimized as well. In fact, much of generative design and topology optimization relies on AI. In this paper, machine learning is used to optimize a waveguide. It is easy to see the designer or engineer of the future more as an architect than a bricklayer. Now, we play with triangles, but later we could raise our abstraction level to direct the process, while the algorithms fill in the right shapes correctly. Really, this is exactly what generative design is and it has been routinely engaged in for over five years.
Conclusion
At this stage, we can’t know what machine learning and AI will do for the world. We cannot see which AI companies and initiatives will make it and find business models in the future, nor can we see just how much funding and revenue they will get. At the moment, however, we believe that AI will have a fundamental impact on the world. Additive as well will be impacted. We probably shouldn’t all fear for our jobs, but our jobs will change. Overall, the abstraction level will be changed. No longer with our noses in the code, we will instead look around at the horizon. AI will be a tool and given that we have a lot of complexity and variation in our manufacturing it may be a fundamentally interesting tool for us to deploy.
Subscribe to Our Email Newsletter
Stay up-to-date on all the latest news from the 3D printing industry and receive information and offers from third party vendors.
Print Services
Upload your 3D Models and get them printed quickly and efficiently.
You May Also Like
The Dental Additive Manufacturing Market Could Nearly Double by 2033, According to AM Research
According to an AM Research report from 2024, the medical device industry, specifically in dentistry, prosthetics, and audiology, is expected to see significant growth as these segments continue to benefit from...
Heating Up: 3D Systems’ Scott Green Discusses 3D Printing’s Potential in the Data Center Industry
The relentless rise of NVIDIA, the steadily increasing pledges of major private and public investments in national infrastructure projects around the world, and the general cultural obsession with AI have...
AM Research Webinar Explores Continuum’s Sustainable Metal Additive Manufacturing Powders
Metal additive manufacturing (AM) powder supplier Continuum Powders is working to develop solutions that empower industries to reduce waste and optimize their resources. An independent life cycle assessment (LCA) of...
3D Printed Footwear Startup Koobz Lands $7.2M in Seed Round
California-based Koobz is focused on reshoring the U.S. footwear supply chain with advanced manufacturing processes, including 3D printing. The startup just announced that it has added $6 million to its...