Using Semi-Supervised Machine Learning in Laser Powder-bed Fusion Fault Detection
Researchers from the University of Liverpool outline their findings regarding the automatic detection of faults in additive manufacturing products in a recently published paper, ‘Automatic fault detection for laser powder-bed fusion using semi-supervised machine learning.’ Their work continues an ongoing trend in perfecting 3D printing and additive manufacturing techniques to enhance numerous industries currently delving into the technology.
The scientists have created a machine learning algorithm, using a semi-supervised approach, to detect AM product flaws. The algorithm draws data from parts that are already certified as well as those with unknown quality. While this not only furthers the quality of 3D printing, the approach is much more efficient and affordable—in relation to laser powder-bed fusion printing.
The researchers compiled data using ‘high precision photodiodes,’ a type of very sensitive sensor able to process measurements to assess quality.
“Understanding the correlations between this data and build quality is a challenging area,” state the researchers. “However, advances in machine learning have made it possible to create and apply intelligent algorithms to large datasets for decision making.”
“Such algorithms can identify patterns in large data, after being trained. The current work is based on the hypothesis that, using large amounts of process measurements from L-PBF machines, machine learning can be used to quickly and cheaply classify the success of L-PBF builds.”
The semi-supervised approach is exactly what it sounds like—a mode in between completely supervised with labeled data and defined sets and unsupervised learning where patterns much be discovered with unlabeled data.
“With a semi-supervised approach, the user provides some labeled data and some unlabeled data at the same time,” state the researchers. “The model may then attempt to establish a decision boundary and classifies the data into clusters; based on the characteristics of the provided labeled and unlabeled information.”
The researchers point out that a semi-supervised approach works well in a scenario rich in unlabeled data, with only a few labeled data—saving time and money in the number of experiments performed.
This is a data-based project, based only on patterns from the photodiode measurements, helping the researchers identify the causes of 3D printing defects. Often, they are the result of poor settings, inferior supports, issues with powder, or temperature or material problems. The four following parameters have the most effect on part quality:
- Part bed temperature
- Laser power
- Scan speed
- Scan spacing
For this study, two L-PBF builds were used, and the researchers built 50 tensile test bars, with 25 yielded in each build. Data was gleaned from each build, as ‘the x and y position of the laser was collected alongside time history measurements from 2 photodiodes sensors (sample frequency equal to 100 kHz, resulting in approximately 400 GB of data per build).’

x–y coordinates of the laser as a single layer of a build is being constructed. Red areas indicate the positions of the 25 tensile test bars while blue represents the laser path. Note that x–y coordinates are calculated from galvanometer measurements and that, for confidentiality reasons, units of position have been left as arbitrary.
Tensile tests were performed by the research time, and each bar was judged as either acceptable or faulty, with a 77 percent test rate.
“The results show that semi-supervised learning is a promising approach for the automatic certification of AM builds that can be implemented at a fraction of the cost currently required,” concluded the researchers.
“Future work aims to investigate whether classification can be improved through the use of additional, complimentary sensing systems (acoustic sensors and thermal imaging cameras, for example).”
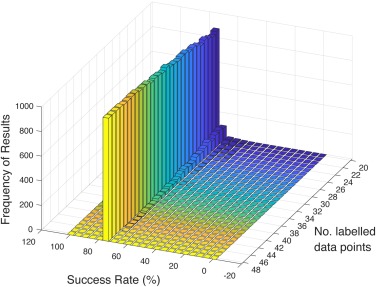
Example semi-supervised learning results. Red and green contours show the inferred geometry of the two Gaussian distributions in the mixture. Circles represent the true labels that were assigned to each specimen, while triangles show the inferred labels.
With the inception of 3D printing came the continual marveling of all we can create—but also continual suspicion regarding whether the parts can hold up for functional use, some of it which is meant to be highly industrial. Testing of parts has become an extensive field on its own, whether in improving CT scanning procedures, testing 3D printed motors for the military, or even using robotics for such purposes. Find out more about testing of parts in relation to laser powder-bed fusion here. What do you think of this news? Let us know your thoughts! Join the discussion of this and other 3D printing topics at 3DPrintBoard.com.
[Source / Images: Automatic fault detection for laser powder-bed fusion using semi-supervised machine learning]Subscribe to Our Email Newsletter
Stay up-to-date on all the latest news from the 3D printing industry and receive information and offers from third party vendors.
Print Services
You May Also Like
3D Printing Grows to $15.9B in 2024 Amid Shifting Industry Dynamics
The global additive manufacturing (AM) market reached $15.9 billion in calendar year 2024, according to “Q4 2024 3DP/AM Market Data and Forecast” from Additive Manufacturing Research (AM Research). Despite a...
3DPOD 247: LJ Holmes, Executive Director for the Center of Advanced Manufacturing and Materials at Harrisburg University
Executive Director for the Center of Advanced Manufacturing and Materials at Harrisburg University, Larry “LJ” Holmes is a pioneer in applying additive manufacturing to defense and other critical sectors. Part...
Thai Startup OsseoLabs to Cut Surgery Time with 3D Printed Magnesium Implants
A patient undergoing mandibular reconstructive surgery typically faces two separate operations: one to place a custom-fit titanium plate and another month later to remove it. But what if that second...
Japanese Advanced Manufacturing Capabilities Grow in Europe with Sodick’s Purchase of Prima Additive
The global economy is currently undergoing a reshuffling in terms of what gets manufactured where. In large part, this trend is being driven by new geopolitical alliances and the need...